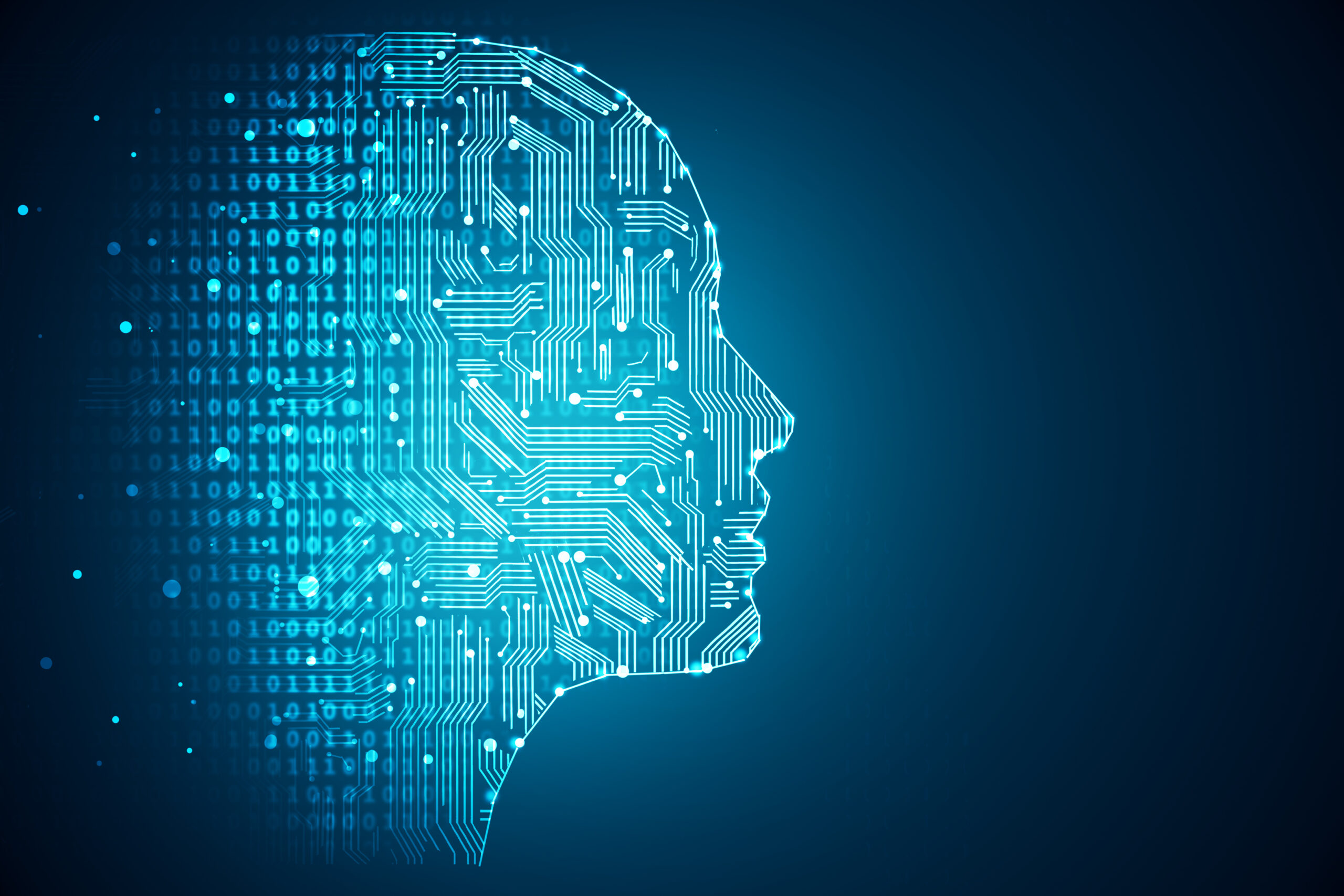
2021 was another bumper year for fines slapped against financial institutions (FIs) for failures in anti-money laundering (AML) compliance.
AML shortcomings in transaction monitoring are a global problem. Countries whose banks were hit with fines include the United States, Germany, the Netherlands, Norway, Latvia, France, the UAE, India, Malaysia, and South Africa. Fines imposed on FIs by regulators could reach as high as $2 billion for a second year running when the final figures come in, according to estimates.
The continuous vigilance of regulators should serve as a wake-up call for financial institutions worldwide to take stock in failures and take action to change the trend in 2022.
Some guilty parties lacked an AML compliance culture or even engaged in outright fraud and corruption. Others turned a blind eye.
For FIs investing in large and costly compliance teams and tools, it’s surely frustrating to be hit with fines of tens of millions of dollars for non-compliance. Some banks fined in 2021 were faulted with poor AML programs including implementation or operating outdated AML systems. As we know well, weak AML controls can open the door for financial crimes.
With the rising number of players in the financial ecosystem and the growing volume of global cross-border payments, the challenges are not going to diminish. Just the opposite.
Financial institutions are surely asking themselves what do to next. Thanks to the advancement of AML technologies based on AI, a direct path has been paved to reverse the trend.
Indeed, 2022 can be the year of change by bringing AI onboard for AML efforts. Here are some ways banks, payment service providers and fintechs can benefit from AML programs powered by AI.
Reduce false alarms
Regulators require financial institutions to submit SARs to cover activity suspected as money laundering, terrorist financing or other criminal offenses such as cybercrime and fraud. They serve as a tool for regulatory and law enforcement bodies.
Failing to submit SARs is a serious violation of AML laws. Many banks took a hit and were fined for this violation in 2021.
FIs operating legacy rules-based systems are inundated with a high volume of false positives, which can reach up to 99% in some cases since they monitor for set thresholds. With so many transactions red-flagged, it’s easy to understand how AML compliance teams would have difficulty deciding which cases warrant referral to financial law enforcement.
Besides being expensive to operate as they require huge manpower, known rules can be easily accessed and outsmarted by bad actors including in cyber-engineered attacks.
Using unsupervised machine-learning AI for transaction monitoring can pinpoint abnormal activity outside normal patterns of financial transactions. In this way, FIs can focus on the cases that really matter, reduce investigations, and avoid slowing down and blocking transactions.
Semi-supervised AI based on known cases of money-laundering can also further train and perfect the detection system.
Fill in missing information
Poor monitoring of customer identities was another factor that caused FIs to miss cases of suspected money-laundering.
In some cases, not enough information is collected at the source to verify the identity of the client. Moreover, KYC data is not visible along the full trail of cross-border money transfers in complex paths when correspondent banks must be involved.
Machine-learning AI-based AML can connect the dots and put together a proxy of a client’s type of identity for compliance teams even when KYC information is missing by creating profiles of pseudo customer activity. With the growing volume of players in the financial system, this ability enabled by AI is becoming increasingly critical.
Manage risk
Conducting customer risk assessment is a necessity to prevent financial institutions from being used, intentionally or unintentionally, for criminal activities. Regulators worldwide are increasingly issuing directives based on the Financial Action Task Force (FATF) guidance to adopt a risk-based approach to AML technologies to improve the speed, quality, and efficiency of AML measures.
Regulators issued many fines in 2021 for inadequate measures to monitor and mitigate money-laundering risks, including handling transactions from countries on sanctions lists.
Using unsupervised AI to analyze risk indicators associated with financial crimes can detect abnormal activity and effectively calculate and pinpoint transactions indicating suspicious activities. This risk-based AI approach can paint a clear picture of the suspected type of crime for compliance teams. By allowing the data to lead to the abnormalities, instead of telling the system what to look for in a rules-only based method, unsupervised AI can also detect new and unpredictable typologies, or “unknown-unknowns.”
AI technologies for AML thereby also reduce risk exposure compared with rules-based systems that are inherently outdated since they are based on known rules and not ready to pounce on new risk typologies that can hit today or tomorrow.